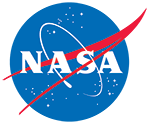
SMAP L2 Radar/Radiometer Half-Orbit 9 km EASE-Grid Soil Moisture, Version 3
Data set id:
SPL2SMAP
DOI: 10.5067/8YTYSV6JGBK2
This is the most recent version of these data.
Version Summary
Version Summary
Changes to this version include:
For the full major and minor version history, go to https://nsidc.org/data/smap/version-history
- Transitioned to Validated-Stage 2
- Uses SPL2SMA V3 Validated and SPL2SMP V3 Validated as input
- Snapshot beta parameter estimation
- Updated soil texture files (renormalized cases where sand+clay > 100%)
- Updated default beta parameter
For the full major and minor version history, go to https://nsidc.org/data/smap/version-history
Overview
This Level-2 (L2) soil moisture product provides estimates of global land surface conditions retrieved by both the Soil Moisture Active Passive (SMAP) radar and radiometer during 6:00 a.m. descending half-orbit passes. SMAP L-band backscatter and brightness temperatures are used to derive soil moisture data, which are then resampled to an Earth-fixed, global, cylindrical 9 km Equal-Area Scalable Earth Grid, Version 2.0 (EASE-Grid 2.0).
Parameter(s):
BRIGHTNESS TEMPERATURESIGMA NAUGHTSOIL MOISTURE
Platform(s):
SMAP
Sensor(s):
SMAP L-BAND RADAR, SMAP L-BAND RADIOMETER
Data Format(s):
HDF5, HDF5
Temporal Coverage:
13 April 2015 to 7 July 2015
Temporal Resolution:
- 49 minute
Spatial Resolution:
- 9 km
- 9 km
Spatial Reference System(s):
WGS 84 / NSIDC EASE-Grid 2.0 Global
EPSG:6933
Spatial Coverage:
N:
85.044
S:
-85.044
E:
180
W:
-180
Blue outlined yellow areas on the map below indicate the spatial coverage for this data set.
Data Access & Tools
A free NASA Earthdata Login account is required to access these data. Learn More
Documentation
User Guide
ATBDs
General Resources
Quality Assessment Reports
Product Specification Documents
Help Articles
General Questions & FAQs
SMAP Ancillary data sets are used to produce SMAP Level-1, -2, -3, and -4 standard data products.
This short article describes the customization services available for SMAP data using Earthdata Search.
How to Articles
Many NSIDC DAAC data sets can be accessed using the NSIDC DAAC's Data Access Tool. This tool provides the ability to search and filter data with spatial and temporal constraints using a map-based interface.Users have the option to
To convert HDF5 files into binary format you will need to use the h5dump utility, which is part of the HDF5 distribution available from the HDF Group. How you install HDF5 depends on your operating system.
The following are instructions on how to import and geolocate SMAP Level-1C HDF5 data in ENVI.
Testing notes
Software: ENVI
Software version: 5.3 and above. If using version 5.3, service pack 5.3.1 is needed.
Platform: Windows 7
Data subscriptions are available for select NSIDC DAAC data collections. Once signed up, the subscription service automatically sends you new data as they are delivered from active NASA satellite missions.
All data from the NASA National Snow and Ice Data Center Distributed Active Archive Center (NSIDC DAAC) can be accessed directly from our HTTPS file system, using wget or curl. Basic command line instructions are provided in the article below.
NASA Earthdata Search is a map-based interface where a user can search for Earth science data, filter results based on spatial and temporal constraints, and order data with customizations including re-formatting, re-projecting, and spatial and parameter subsetting.