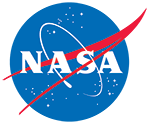
IceBridge-Related DMS-Derived L4 Sea Ice Surface Cover Classification Orthorectified Images, Version 1
Data set id:
RDSISCO4
DOI: 10.5067/1LI57H56EB7G
This is the most recent version of these data.
Version Summary
Version Summary
Initial release
Overview
This data set contains reprocessed, orthorectified images depicting labels that indicate the sea ice surface category, created by processing IceBridge DMS L0 Raw Imagery with the Open Source Sea-ice Processing Algorithm. Orthorectification was done using digital elevation models from the IceBridge DMS L3 Ames Stereo Pipeline Photogrammetric DEM collection. The standard (non-orthorectified) images are available as IceBridge-Related DMS-Derived L4 Sea Ice Surface Cover Classification Images.
Parameter(s):
SEA ICE/OCEAN CLASSIFICATION
Platform(s):
C-130, DC-8, HU-25A, HU-25C, P-3B, WP-3D ORION
Sensor(s):
DMS
Data Format(s):
GeoTIFF
Temporal Coverage:
23 March 2010 to 25 July 2017
Temporal Resolution:
- Varies
Spatial Resolution:
- varies
- varies
Spatial Reference System(s):
WGS 84 / NSIDC Sea Ice Polar Stereographic North
EPSG:3413
Spatial Coverage:
N:
90
S:
60
E:
180
W:
-180
Blue outlined yellow areas on the map below indicate the spatial coverage for this data set.
Data Access & Tools
A free NASA Earthdata Login account is required to access these data. Learn More
Documentation
Help Articles
How to Articles
Many NSIDC DAAC data sets can be accessed using the NSIDC DAAC's Data Access Tool. This tool provides the ability to search and filter data with spatial and temporal constraints using a map-based interface.Users have the option to