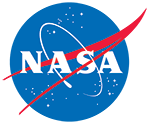
AMSR-E/AMSR2 Unified L3 Global Monthly 25 km EASE-Grid Snow Water Equivalent, Version 1
Data set id:
AU_MoSno
DOI: 10.5067/43NH9LHM9YRK
This is the most recent version of these data.
Version Summary
Version Summary
Initial data release
Overview
This AMSR-E/AMSR2 Unified Level-3 (L3) data set provides monthly mean estimates of Snow Water Equivalent (SWE). SWE was derived from brightness temperature measurements acquired by the Advanced Microwave Scanning Radiometer 2 (AMSR2) instrument on board the JAXA GCOM-W1 satellite.
The SWE data is rendered to an azimuthal 25 km Equal-Area Scalable Earth Grid (EASE-Grid) for both the Northern and Southern Hemisphere.
Note: This data set uses JAXA AMSR2 Level-1R (L1R) input brightness temperatures that are calibrated, or unified, across the JAXA AMSR-E and JAXA AMSR2 L1R products.
Parameter(s):
SNOW WATER EQUIVALENT
Platform(s):
GCOM-W1
Sensor(s):
AMSR2
Data Format(s):
HDF-EOS5
Temporal Coverage:
2 July 2012 to present
Temporal Resolution:
- 1 month
Spatial Resolution:
- 25 km
- 25 km
Spatial Reference System(s):
NSIDC EASE-Grid North
EPSG:3408
NSIDC EASE-Grid South
EPSG:3409
Spatial Coverage:
N:
90
S:
-90
E:
180
W:
-180
Blue outlined yellow areas on the map below indicate the spatial coverage for this data set.
Data Access & Tools
A free NASA Earthdata Login account is required to access these data. Learn More
Documentation
Help Articles
How to Articles
Many NSIDC DAAC data sets can be accessed using the NSIDC DAAC's Data Access Tool. This tool provides the ability to search and filter data with spatial and temporal constraints using a map-based interface.Users have the option to
To convert HDF5 files into binary format you will need to use the h5dump utility, which is part of the HDF5 distribution available from the HDF Group. How you install HDF5 depends on your operating system.
Data subscriptions are available for select NSIDC DAAC data collections. Once signed up, the subscription service automatically sends you new data as they are delivered from active NASA satellite missions.
All data from the NASA National Snow and Ice Data Center Distributed Active Archive Center (NSIDC DAAC) is directly accessible through our HTTPS file system using Wget or curl. This article provides basic command line instructions for accessing data using this method.