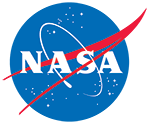
AMSR-E/Aqua Monthly L3 5x5 deg Rainfall Accumulations, Version 2
Data set id:
AE_RnGd
DOI: 10.5067/AMSR-E/AE_RNGD.002
This is the most recent version of these data.
Version Summary
Version Summary
Overview
This Level-3 rainfall accumulation product (AE_RnGd) consists of two grids of 28 rows by 72 columns of monthly averaged rainfall accumulation over ocean and land. Both grids are 5 degree by 5 degree resolution.
Parameter(s):
PRECIPITATION AMOUNTRAIN
Platform(s):
AQUA
Sensor(s):
AMSR-E
Data Format(s):
HDF-EOS2
Temporal Coverage:
19 June 2002 to 1 October 2011
Temporal Resolution:
- 1 month
Spatial Resolution:
- 5 deg
- 5 deg
Spatial Coverage:
N:
70
S:
-70
E:
180
W:
-180
Blue outlined yellow areas on the map below indicate the spatial coverage for this data set.
Data Access & Tools
A free NASA Earthdata Login account is required to access these data. Learn More
Documentation
User Guide
ATBDs
General Resources
Quality Assessment Reports
Help Articles
General Questions & FAQs
This article describes what customizing services are available for AMSR-E data using Earthdata Search.
How to Articles
Many NSIDC DAAC data sets can be accessed using the NSIDC DAAC's Data Access Tool. This tool provides the ability to search and filter data with spatial and temporal constraints using a map-based interface.Users have the option to
To convert HDF5 files into binary format you will need to use the h5dump utility, which is part of the HDF5 distribution available from the HDF Group. How you install HDF5 depends on your operating system.
All data from the NASA National Snow and Ice Data Center Distributed Active Archive Center (NSIDC DAAC) can be accessed directly from our HTTPS file system, using wget or curl. Basic command line instructions are provided in the article below.
NASA Earthdata Search is a map-based interface where a user can search for Earth science data, filter results based on spatial and temporal constraints, and order data with customizations including re-formatting, re-projecting, and spatial and parameter subsetting.